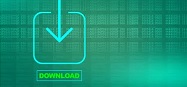

Next, several template-based segmentations, for example, genetic-algorithm-based template matching and 3D template matching, create template models to detect the target position of the nodules. Indeed, it is difficult due to the fuzzy nature of the lungs and nodules. Regrettably, these methods require a proper choice of the initial region and homogeneity criteria for accurate segmentation. To segment the ROI, they identify an initial region and determine the homogeneity between neighbouring pixels. Unlike the intensity-based methods, region-based segmentation schemes consider not only spatial information, but also the relationship between the pixels. Moreover, they are less sensitive to noises and blur boundaries because they neglect spatial information about the image. While they are easy to use, the effectiveness of these methods strongly depends on the predefined or initial threshold values. Nodule detections using fixed thresholding, Otsu’s thresholding, and optimal thresholding are examples of the intensity-based methods. They segment the region of interest (ROI) by grouping image pixels based on one or more predefined grey-value. Intensity-based segmentations are the fastest and simplest methods. The existing segmentation methods for this step can be categorized into four major groups: intensity-based, region-based, template-based, and machine-learning-based methods. Afterwards, potential nodules scattered in the segmented lungs are extracted. In this step, the lung regions from the input CT scan are initially segmented. The first step aims to extract the lesions that have a high possibility of being a nodule. Generally, most of these works are based on two fundamental steps: (i) suspicious nodule detection and (ii) false reduction. Additionally, they can make the interpretation process faster and more convenient by stacking the multiple sequential CT slices together as volumetric images.ĭifferent research on automated nodule detections have emerged over the years.
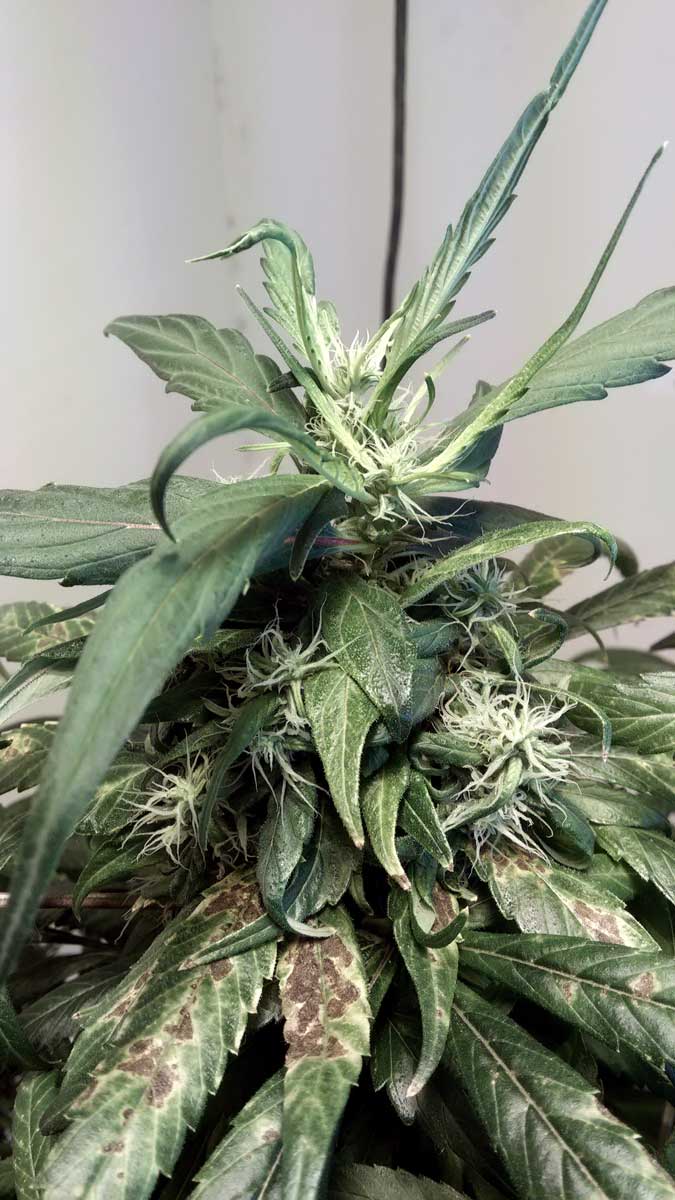
They were developed with aim of providing second options for better, more confident, and reliable diagnosis results. For these reasons, automated nodule detection schemes were strongly demanded as supportive tools. Moreover, this task requires a high proficiency by the interpreters as an incorrect or miss-detection of the potential malignant nodule is highly-risky for patients. It is effort-intensive and error-prone because the interpreter has to read the massive amount of CT scans slice-by-slice.
#FIREFLY LEAF ON THE WIND HOLE IN CHEST MANUAL#
With the increasing number of lung cancer cases, manual detection of nodules has become a challenging task. There are several clinical factors to distinguish benign and malignant pulmonary nodules, such as size, location, calcification, margin-types, and texture. However, malignant nodules are cancerous and can even metastasize to other parts of the body. Benign nodules are commonly found in almost all CT examinations, but they are not cancer. They can be categorized into two types, namely, benign and malignant. However, not all the nodules discovered in the lungs are cancerous. They form due to the inflammation of pulmonary structures, and often react to infection and diseases. Nodules are generally regarded as an early signal of lung cancer. They are the round or oval-shaped opacities on the CT scans that have well-defined or irregular margins. CT can provide detail morphologic information about lung cancer by detecting abnormal lesions inside the lungs, called pulmonary nodules.
#FIREFLY LEAF ON THE WIND HOLE IN CHEST TRIAL#
According to the National Lung Screening Trial (NLST), the mortality rate of lung cancer reduced up to 15%–20% in patients who performed low-dose CT screening. Importantly, since the advent of medical imaging technologies, computed tomography (CT) became an effective modality for the early diagnosis of lung cancer.
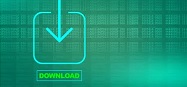